Important Management Practices Driven by Data Science
IN VIEW OF THE UNCERTAINTIES IN DECISION MAKING, THERE ARE ALWAYS ELEMENTS OF RISKS INVOLVED IN EVERY DECISION TAKEN BY THE MANAGER.
USE OF DATA SCIENCE IN DECISION MAKING
The objective of management is to minimize risk. To bring this to fruition and enhance the robustness of a decision, most organizations tend to use relevant data to analyse the challenge before arriving at an optimum solution. For example, by collecting data on the financial discipline of an entrepreneur, and industry growth of the relevant industry, the bank loan manager can estimate risk. If the risk is very high, the manager may reject the loan, and vice versa, thus minimizing the overall risk for the organization. Similarly, by collecting various data such as historical demand data of different products, order fulfilment data of the vendors, failure data of equipment, the PPPM can estimate the risk involved in a production plan, and take a decision accordingly. Thus, a data driven decision-making approach would help understand and reduce the risk for a business. Below, we outline a few examples of data driven approaches and their advantages.
DATA SCIENCE DRIVEN APPROACH IN MANAGING NPAS IN BANKS:
Many banks report large Non-Performing Assets (NPAs) mainly due to bad loans (see [4]). Some of the common problems of NPA management include highly divergent loan appraisal standards, absence of a common database, absence of timely intervention, excessive use of discretion, reliance on business models with suspect assumptions etc. Data driven management systems with a common big data platform - which takes, ingests and processes data related to a business from various sources, including social media, and deploys analytical tools to create effective dashboards with warning signals - can help monitor and take appropriate actions on loans. Also, the use of artificial intelligence driven rule-based tools can help eliminate or reduce subjectivities in appraisals and human errors in assessments, through validations and logical extrapolations, thus reducing the possible number of bad loans converting to NPAs.
DATA SCIENCE DRIVEN APPROACH IN MANAGING INVENTORY:
In many companies, inventory cost is one of the major costs, which accounts for about 10-20% of sales. Inventory is the quantity of finished products and raw materials that companies keep in stock with a view to sell them or use them for uninterrupted production/manufacturing as and when needed. Inventory managers work towards maintaining the right amounts of products in inventory, since keeping large units in inventory would lead to a huge amount of unutilized working capital, whereas keeping less units in the warehouse may leave them out of stock and lead to loss of sales and customer dissatisfaction. As an example, consider a phenomenon that occurred in Big Bazaar in 2006 (see [5]) when they announced a “Sabse Sasta Din” on January 26, with huge discounts on many items including televisions, refrigerators, food items etc. All the stake holders from marketing, operations, IT and category managers had jointly planned for this event, and worked with several vendors across India to stock up on a good number of items in Big Bazaar outlets. The executives estimated and hoped that this event would trigger higher sales and clear the items they had specially procured for the event. However, what happened was totally different. Each store witnessed a turn-out in such huge numbers that the police had to be summoned to manage crowds. Moreover, they ran out of stocks. Many of their regular customers went home dissatisfied as they had gone through a lot of trouble to enter the store, only to realise that the product they wished to purchase was out of stock. Big Bazaar had to close down their stores by afternoon, and announce that the “Sabse Sasta Din” sale would continue for the next 2 days as well. By quickly procuring more items, they ran the event over the next two days, and realized record sales. It is here that they underestimated demand for the items at the price discounts offered during the event.
There are several factors, such as price, season, competitor/ substitute products etc., that influence the demand. It is important to collect data and estimate the effect of each of these factors effectively so as to estimate the demand and plan inventory accordingly. To see how Data Science can help in inventory planning, let’s look at an example of another retailer based in Europe, who was challenged by high inventory cost. The retailer had an inventory cost of 22% of sales, and also had a huge loss of sales. The analytics experts studied the profile of products in stock, and observed that large items in inventory are those which have competing products in the market, and the only way they could compete in the market was through price discounts. Also, it was noticed that the store ran out of stock for some of the essential products when the customers needed them, leading to loss of sales. The experts recommended an increase in the safety stock of such items. Based on the recommendation, the retailer announced price discounts on select items. This helped them realize an increased demand for these products in a short period of time. At this point of time, they developed an analytical demand planning solution using statistical and machine learning algorithms by taking into account the data of demand, store footfall, customer ratings of products (web-based ratings), marketing variables, weather, etc. This resulted in an improvement of demand forecast accuracy from 40% to 60%, leading to an inventory reduction of 42%, reducing the stock out situation by 50% from its existing level. All of this led to an overall cost savings amounting to about 10% of total sales.
DATA SCIENCE DRIVEN APPROACH IN MANAGING EQUIPMENT FAILURE:
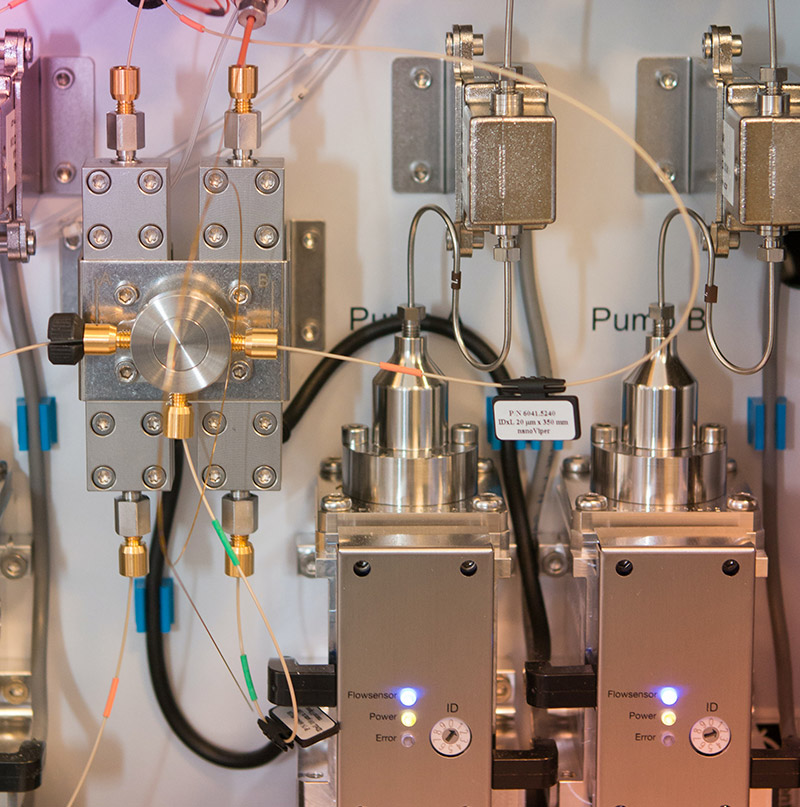
DATA DRIVEN ORGANIZATIONS AND THEIR ADVANTAGES:
ALL OF US WOULD HAVE RECEIVED A PRODUCT RECOMMENDATION WHILE VISITING THE AMAZON WEBSITE OR THROUGH EMAIL. THIS IS A TYPICAL EXAMPLE OF A DATA DRIVEN BUSINESS DECISION.
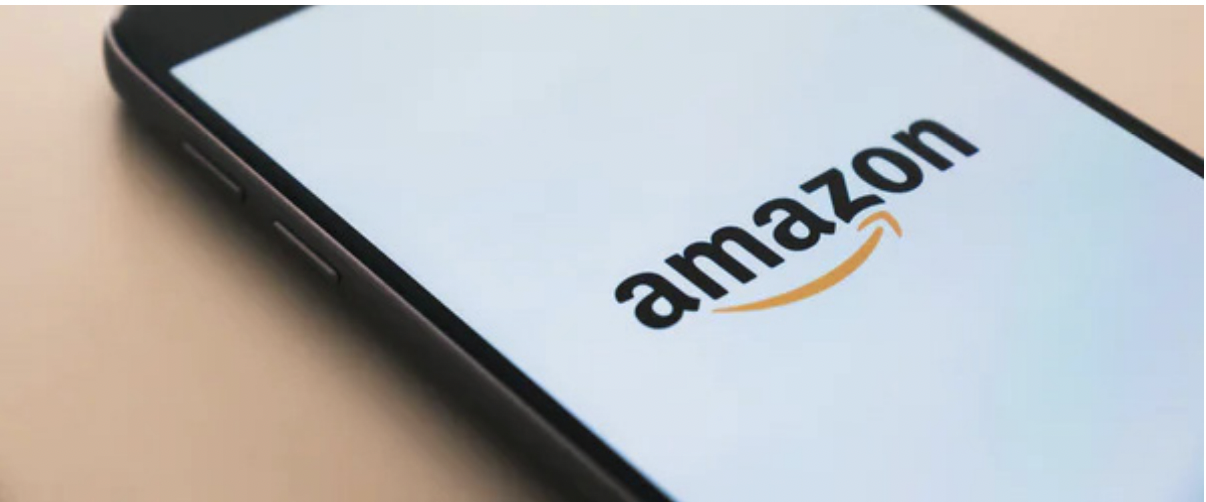
IMPORTANT STEPS IN CREATING A DATA SCIENCE DRIVEN ORGANIZATION
i) Understand the Business and Decision-Making Process: For most organizations, lack of data is not a problem. In fact, it’s the opposite: there is often too much information available to make a clear decision. With so much data to sort through, organizations need to have a clear understanding of their business decision-making process and a better data science strategy to support that process. For example, to promote a product, the use of data on customer segments and product preferences as observed in product reviews.
ii) Establish Performance Metrics: In order to successfully translate this vision and business goals into actionable results, the next step is to establish clear performance metrics. The metrics is defined considering each business unit’s objective and designed to improve their performance.
iii) Architect the End-to-End Solution: Organizations need to think more organically about the end-to-end data flow and architecture that will support their data science solutions. Data architecture is the process of planning the collection of data, including the definition of the information to be collected, the standards and norms that will be used for its structuring and the tools used in the extraction, storage and processing of such data. This stage is fundamental for any project that performs data analysis, as it is what guarantees the availability and integrity of the information that will be explored in the future. It can be said that at this point there is an intersection of the technical and strategic visions of the project, as the purpose of this planning task is to keep the data extraction and manipulation processes aligned with the objectives of the business.
iv) Build Data Science Tool Box: Based on the requirements, the organization needs to develop toolkits such as demand forecasting, marketing, human resource planning, warranty planning, spare parts planning, predictive asset maintenance, financial planning etc. Since all these tools use commonly available data on one platform, it will be easy for all the stake holders to trust the solution developed in this manner.
v) Unify Your Organization’s Data Science Vision: Develop interactive dashboards which provide a unified view of all the aspects of the organization. The advantage of such a tool is to understand how a change in any particular function affects other functions, and hence leads to a decision which is easily acceptable by all functions and easily implementable as well. For example, if the marketing department complements a promotion (price discount), this would impact revenue, and would also involve increased inventory, and probably increased production as well. Using the unified tool, one can visualize the production and storage constraints, and then take appropriate decisions collectively.
vi) Keep Humans in the Loop: One of the most important steps is to ensure that the human inputs are considered at each step of the process. For example, the Data Science tool may recommend the reduction of price with reduced features for a product considering external market related data. However, the company’s brand or reputation may be sacrificed if they offer a flagship product at a lower price with reduced features. Human intervention is an important input to be considered to effectively fine tune the recommendation and implement it in the organization.
CONCLUSION
The success of an organization depends heavily on the decisions taken by the management of the company at various levels. Every organization will have different departments/functions, and the decisions taken by managers of each department depends on the availability and visibility of data. However, these decisions taken by one department could heavily impact other departments, and hence the overall company’s growth and profitability. By following a unified data science approach and having an organizational culture of data driven decision-making process, as explained in this article, companies would be able to achieve higher growth and profitability in an efficient manner.